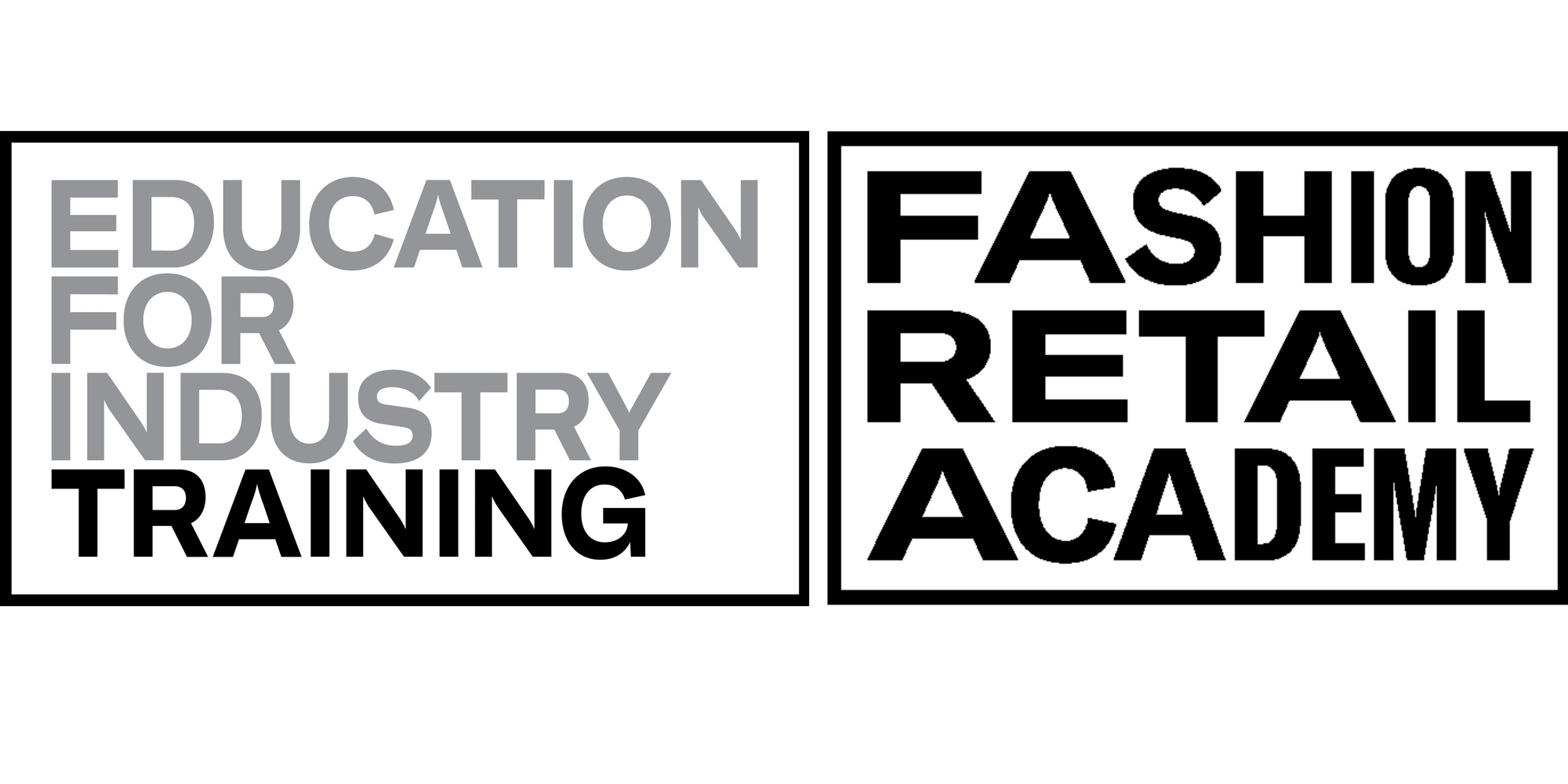
FV | The Data Adventure of Sam & Alex
FRA Online Courses
Created on January 9, 2024
More creations to inspire you
Transcript
The Data Adventure of Sam & Alex
Start
Once upon a time, in the busy luxury fashion company of Datachan, there worked two colleagues, Sam and Alex. Alex was a visual merchandiser who loved to explore the vast world of unstructured narratives and stories. Sam was a meticulous fashion business accountant who thrived on neatly organized spreadsheets and structured data.
Click on the first story to continue.
The Structure Dilemma & Unstructured Odyssey
One day, the owner of Datachan approached Sam and Alex with a unique challenge. The company was facing a surge of unstructured and structured data, and they needed to find ways to make sense of it all.
Click the next button to the right middle of this page to continue.
Sam, armed with their trusty calculator, dove into the structured data world.
Alex embarked on an unstructured odyssey, exploring the vast realm of social media posts, customer reviews, and creative content.
The Difference?
Structured Data
Unstructured Data
Click each button to read more.
As the two friends delved deeper into their respective data realms, they realised the true power lay in collaboration. Sam's structured insights complemented Alex's unstructured narratives. Together, they could paint a comprehensive picture of Datachan's current state and future possibilities.
Instructions:1. Recap now on the definitions carefully by clicking on the definitions button below.2. On the next page, determine whether the example belongs to the world of structured data, unstructured data, or perhaps even the enigmatic world of semi-structured data.3. Drag each example into the appropriate category.4. Click the Check button to see your asnwers.What are you waiting for? Let’s embark on this data journey!Click next to start.
Welcome, data explorers!
In this sorting activity challenge, we’ll help Alex and Sam as we categorise examples of data into the structured and unstructured data worlds.
Definitions
Drag each example at the bottom into its category at the top. Once done, click Check to see your results.
Click on the second story to continue.
As Sam and Alex delved further into their data quest, they stumbled upon a mysterious artefact called the 'Data Campass'. Legend had it that this compass could reveal the secrets of data's origin and purpose, an extremely important part to recognise and classify data.
The Data Compass: Source and Use Revelation
TheContextual Enigma
The Data Source
The Quality Conundrum
The Analysis Oracle
Begin by clicking the first button, "Structured Data Source." Then, come back to the Compass by clicking Return. Each time you do this, the next button will become available. Finally, on the last page, the Continue button will become available.
Structured Data Source
Unstructured Data Source
Structured Data Context
Unstructured Data Context
Structured Data Quality
Unstructured Data Quality
Structured Data Analysis
Unstructured Data Analysis
Back to Compass
Structured Data Source
Structured data has its roots in internal databases and ERP systems, the systems that administrative departments usually meticulously maintain.
TheContextual Enigma
The Data Source
The Quality Conundrum
The Analysis Oracle
Structured Data Source
Unstructured Data Source
Structured Data Context
Unstructured Data Context
Structured Data Quality
Unstructured Data Quality
Structured Data Analysis
Unstructured Data Analysis
Back to Compass
Unstructured Data Source
Unstructured data, however, flows in from other sources like social media APIs, web scraping, and the vast wilderness of colleagues’ qualitative research.
TheContextual Enigma
The Data Source
The Quality Conundrum
The Analysis Oracle
Structured Data Source
Unstructured Data Source
Structured Data Context
Unstructured Data Context
Structured Data Quality
Unstructured Data Quality
Structured Data Analysis
Unstructured Data Analysis
Back to Compass
Structured Data Analysis
Structured data analysis involve using structured query languages (SQL), statistical methods, and business intelligence tools to extract insights from organised datasets with a predefined structure, such as tables in relational databases. Why is it important for you and the organisation you work for to know about it?
Click each button to read more.
Efficient Querying
Statistical Analysis
Business Intelligence (BI)
Structured Querying Languages (SQL)
Aggregated Insights
Predictive Modeling
Database Management
Ad Hoc Queries
Ease of Integration
Regulatory Compliance
Structured Reporting
TheContextual Enigma
The Data Source
The Quality Conundrum
The Analysis Oracle
Structured Data Source
Unstructured Data Source
Structured Data Context
Unstructured Data Context
Structured Data Quality
Unstructured Data Quality
Structured Data Analysis
Unstructured Data Analysis
Back to Compass
Unstructured Data Analysis
Unstructured data analysis involves employing advanced techniques, such as NLP and image recognition, to extract insights from diverse data forms like text, images, audio, and video.Why is it important for you and the organisation you work for to know about it?
Click each button to read more.
Extracting Meaningful Insight
Enhancing Decision Making
Advanced NLP and Language Processing
Image and Video Understanding
Innovation and Creativity
Adapting to Diverse Data Formats
Interdisciplinary Skills Development
Enhancing Communication Skills
Ethical Considerations
TheContextual Enigma
The Data Source
The Quality Conundrum
The Analysis Oracle
Structured Data Source
Unstructured Data Source
Structured Data Context
Unstructured Data Context
Structured Data Quality
Unstructured Data Quality
Structured Data Analysis
Unstructured Data Analysis
Back to Compass
Structured data analysis involves using structured query languages (SQL), statistical methods, and business intelligence tools to extract insights from organised datasets with a predefined structure, such as tables in relational databases. It is important for you to understand structured data context because it guides the design of database systems, informs data analysis approaches, and ensures that data is used effectively to meet organisational goals. The context provides the foundation for making informed decisions about how to store, manage and extract values from structured data.
Structured Data Context
Click each button to read more.
Organised Storage and Retrieval
Consistency and Data Integrity
Querying and Analysis
Common Examples and Use Cases
Data Relationships
Schema Definition
Ease of Integration
Efficient Processing
TheContextual Enigma
The Data Source
The Quality Conundrum
The Analysis Oracle
Structured Data Source
Unstructured Data Source
Structured Data Context
Unstructured Data Context
Structured Data Quality
Unstructured Data Quality
Structured Data Analysis
Unstructured Data Analysis
The context of unstructured data refers to the environment, characteristics, and challenges associated with data that lacks a predefined organisational structure. It is essential for you to understand unstructured data context for developing effective strategies in data management, preprocessing, and analysis. The unstructured data context will guide your selection of appropriate tools, technologies, and methodologies to derive valuable insights from the diverse and dynamic nature of unstructured data sources. Recognising the challenges inherent to unstructured data will help you address issues related to data quality, privacy, and ethical considerations during the analysis process.
Back to Compass
Unstructured Data Context
Click each button to read more.
Diverse Data Types
No Fixed Schema
Volume and Velocity
Complexity and Lack of Organisation
Semantic Ambiguity
Analysis Tecniques
Varied Sources
Dynamic Nature
TheContextual Enigma
The Data Source
The Quality Conundrum
The Analysis Oracle
Structured Data Source
Unstructured Data Source
Structured Data Context
Unstructured Data Context
Structured Data Quality
Unstructured Data Quality
Structured Data Analysis
Unstructured Data Analysis
Back to Compass
Structured data quality refers to the accuracy, completeness, consistency, and reliability of data. It involves ensuring that data stored in databases or structured formats meets specific standards and is free from errors or discrepancies.
Structured Data Quality
Click each button to read more.
Accurate Decision Making
Effective Analysis
Operational Efficiency
Data Integrity
Compliance and Governance
Customer Trust
Efficient Reporting
Interoperability
Reduced Costs
Improved Customer Experience
Data-driven Innovation
TheContextual Enigma
The Data Source
The Quality Conundrum
The Analysis Oracle
Structured Data Source
Unstructured Data Source
Structured Data Context
Unstructured Data Context
Structured Data Quality
Unstructured Data Quality
Structured Data Analysis
Unstructured Data Analysis
Back to Compass
Unstructured Data Quality
Unstructured data quality refers to the accuracy, relevance, and reliability of data that needs a predefined organisational structure. It involves ensuring that unstructured data is meaningful, contextually accurate, and suitable for analysis.
Click each button to read more. Once done, click next to continue.
Insightful Analysis
Informed Decision Making
Effective NLP
Enhanced Image and Video Processing
Comprehensive Data Exploration
Ethical Considerations
Improved Speech Recognition
Meaningful Content Discovery
Relevance in Social Media Analysis
Contextual Understanding
Optimised Machine Learning Models
rmed with the insights from the Data Compass, Sam and Alex could now trace data origins, comprehend its context, evaluate its quality and foresee its analytical destiny. As they shared this newfound wisdom with the CEO, Sam and Alex's adventure became a beacon, forever reminding their colleagues that knowledge about the origins, purpose and maintenance was the key to harnessing data's true power.
A
The Solo Fashion Data Quest
1. Explore a dataset within your own fashion (or otherwise related) organisation or a simulated fashion company.2. Identify the source of the dataset.
You are now asked to delved on your own into a Fashion Data Quest. This solo expedition allows you to explore the world of fashion data at your own pace.
The Fashion Data Quest:
Fashion Data Source & Utilisation Report:
Quest Reflective Analysis:
On completion, reflect on the experience of being solo in this fashion data quest. What challenges did you encounter, and how did you overcome them? Did your independent exploration yield unique insights into the world of fashion data?
Prepare a detailed report focusing on data sources and utilisation within the fashion organisation:1. Identify the Source.2. Analyse the purpose for which the fashion data is collected and how it is utilised within the organisation.3. Share your findings on the Canvas discussion, read and comment the reports of your peers. Extra instructions in support of this activity are provided on the Canvas Discussion spot.
Canvas Discussion
Canvas Discussion Spot: The Solo Fashion Data Quest
Click on the third story to continue.
One day, as Sam and Alex ventured deeper into the heart of Datachan, they encountered a mystical divide known as the "Quantitative and Qualitative Nexus." Here, the Data Compass shimmered with a duality of numerical precision and interpretive richness, leading them to explore the realms of quantitative and qualitative data.
The Symphony of Quantitative & Qualitative Data Realms
Quantitative Data
Qualitative Data
Vs.
Quantitative VS. Structured
Qualitative VS. Unstructured
Data Type
Precision and Accuracy
Analysis Techniques
Data Visualization
Data Integration
Time and Resources
Decision Making
Structured Data
Definition: Structured data refers to information that is organized in a predefined and systematic way. It typically fits neatly into tables or relational databases with rows and columns.Characteristics: Each data element has a clear and well-defined format.Examples: Excel spreadsheets, relational databases, CSV files, tables.
Quantitative data
Definition: Quantitative data refers to information that is numeric and can be measured and expressed with numerical values. It involves quantities and is suitable for mathematical and statistical analysis.Characteristics: Quantitative data is often collected through methods such as surveys, experiments, or measurements. It allows for quantitative comparisons and statistical analysis. Examples: Height and weight measurements, survey responses on a numerical scale.
Unstructured Data
Definition: Unstructured data refers to information that does not have a predefined data model or is not organized in a structured manner.Characteristics: This type of data does not fit neatly into traditional relational databases with rows and columns. Unstructured data can be diverse and may include text, images, audio, video, social media posts, emails, and more.Examples: Text documents, images, audio recordings, video files, emails, social media posts.
Qualitative data
Definition: Qualitative data refers to non-numeric information that provides insights into qualities, attributes, or characteristics. It focuses on understanding the underlying meanings, patterns, and contexts.Characteristics: Qualitative data often involves subjective interpretation and may be collected through methods such as interviews, focus groups, observations, or open-ended surveys. It can include textual information, images, audio, or video, and the emphasis is on capturing the richness and complexity of human experiences.Examples: Interview transcripts, open-ended survey responses, field notes, images, audio recordings.
2
3
4
1
Examples
Read all four examples to continue with this course.
1
2
3
4
Unstructured with Qualitative
A collection of customer reviews obtained from various sources, such as online forums, social media, or product review websites.
Read all four examples to continue with this course.
1
2
3
4
Structured with Quantitative
A simple dataset representing sales data for Datachan.
Read all four examples to continue with this course.
1
2
3
4
Unstructured with Quantitative
Quantitative information only comes in a structured format.There is no example of unstructured data with quantitative information.
Read all four examples to continue with this course.
1
2
3
4
Structured with Qualitative
Unstructured data typically doesn't fit neatly into rows and columns, but for the sake of this example, let's consider a somewhat simplified example of unstructured data with quantitative information. Imagine a collection of customer feedback comments gathered from various sources, where each comment contains both qualitative and quantitative elements:
Read all four examples to continue with this course.
From structured databases filled with quantitative data to unstructured narratives providing qualitative depth, the company’s understanding of its data landscape became richer and more nuanced.
With the knowledge of the Quantitative and Qualitative Data Realms, Sam and Alex returned to Datachan.
Final Quiz
Welcome to the Fashion Data Last Voyage.
This is the last adventure of this book, and you can now now test your understanding. After the quiz, reflect on any challenges you encountered during this week's learning experience. You will be able to find a link to extra resources in the reflection page.
Welcome to the Fashion Data Last Voyage.
Welcome to the Fashion Data Last Voyage.
Welcome to the Fashion Data Last Voyage.
Welcome to the Fashion Data Last Voyage.
Welcome to the Fashion Data Last Voyage.
Welcome to the Fashion Data Last Voyage.
Welcome to the Fashion Data Last Voyage.
Welcome to the Fashion Data Last Voyage.
Now, let’s reflect on the learning during this week. You can share your reflection on this Canvas discussion: During the reflection, you may use the following questions as prompts. Were there any concepts that felt particularly tricky to understand? What strategies did you employ to distinguish between the types of data?
If you would like some extra content to review this material, access a list of suggested content by clicking the icon to the left.
Welcome to the Fashion Data Last Voyage.
Thank you
Structured data refers to the organisation and format of the data, typically seen in tables or databases.Structured data can contain both quantitative and qualitative information in a well-organized format.
Structured
Quantitative
VS
Qualitative data refers to non-numeric information and can be unstructured, structured, or semi-structured.The difference between qualitative and unstructured data is the focus is of the information rather than its organization.
Structured data maintains consistency through predefined rules, formats, and integrity constraints.Ensures that the data is accurate, reliable, and adheres to a set of rules, minimising errors and discrepancies in the information.
Consistency and Data Integrity
Unstructured data analysis fosters innovation and creativity as analysts explore unconventional data sources and develop novel approaches to understanding complex information.
Innovation and Creativity
Structured data can be easily integrated into various software applications and systems facilitating business processes and the collection of analytics.
Ease of Integration
Accurate unstructured data quality is crucial for successful NLP applications, sentiment analysis, and language understanding, enhancing the capabilities of language-based technologies.
Effective NLP
Structured and numeric, suitable for mathematical and statistical analysis. It includes variables that can be measured and counted.
Quantitative
Often unstructured and non-numeric, qualitative data are more challenging to analyse. It includes textual information, images, and other non-numeric formats.
Qualitative
VS
Quantitative data can be easily integrated and analyzed alongside other quantitative datasets for a more comprehensive understanding.
Quantitative
Qualitative data may be challenging to integrate with quantitative data due to differences in format and structure.
Qualitative
VS
Structured data quality safeguards the integrity of databases, maintaining the accuracy and reliability of data over time and preventing data corruption.
Data Integrity
Structured data analysis is crucial for managing databases effectively, ensuring data consistency, integrity, and optimal performance.
Database Management
Quantitative data supports data-driven decision-making by providing numerical evidence and trends.
Quantitative
Qualitative data helps decision-making by providing context, insights, and a deeper understanding.
Qualitative
VS
Quality structured data simplifies and accelerates the process of generating reports. Accurate data ensures that reports provide a true representation of the organization's performance.
Efficient Reporting
In this example, the primary focus is on structured data (Satisfaction Rating), the inclusion of the "Feedback" column introduces qualitative information in a structured dataset. This structured approach allows for easier organisation, retrieval, and analysis of both quantitative and qualitative aspects of customer responses.
Unstructured Data
Alex found herself in a sea of diverse information with no clear structure. Through the power of natural language processing and sentiment analysis, Alex deciphered the emotions and opinions hidden within the unstructured data. This insight proved invaluable for understanding customers’ sentiment, preferences, and fashion trends.
Unstructured data analysis allows for the extraction of meaningful insights from non-traditional data sources, uncovering hidden patterns and trends.
Extracting Meaningful Insights
Structured data is stored in well-organized formats with clearly defined schemas and relationships.This organization enables efficient storage and retrieval of information, making it easy to locate and access specific data when needed.
Organised Storage and Retrieval
Techniques for analyzing images and videos enable a richer understanding of visual data, supporting applications like facial recognition and content moderation.
Image and Video Understanding
High-quality unstructured data is vital for training accurate and reliable machine learning models, enhancing the performance of models that leverage natural language understanding, image recognition, and audio processing.
Optimised Machine Learning Models
Accurate and consistent structured data enhances operational efficiency by reducing the likelihood of errors, minimizing rework, and streamlining business processes.
Operational Efficiency
Structured data follows a predefined schema that outlines the structure, data types, and relationships within the dataset. The schema provides a blueprint for how data is organised, helping users understand the data structure and meaning.
Schema Definition
Well-maintained structured data supports interoperability between different systems and applications. It enables seamless data exchange and integration across the organization.
Interoperability
Structured data examples include tables in relational databases, spreadsheets, and organised datasets.Understanding common use cases helps in choosing appropriate tools and techniques for managing and processing structured data efficiently.
Common Examples and Use Cases
Unstructured data encompasses various data types, including text, images, audio, video, and more.Understanding this diversity is essential for choosing appropriate tools and methods to analyse and extract meaningful insights from different data formats.
Diverse Data Types
In this video, Bernard Marr explains the difference between structured and unstructured data, and why it is important for you to know them.
Accurate unstructured data quality is critical for social media analytics, sentiment tracking, and understanding public opinions, providing valuable insights for businesses and organisations.
Relevance in Social Media Analysis
Quality structured data supports efficient data analysis, enabling organizations to derive meaningful insights, identify trends, and make informed decisions.
Effective Analysis
Structured data analysis supports easy integration with other systems and applications, facilitating data exchange and interoperability.
Ease of Integration
Analysis of structured data supports the creation of structured reports that present information in an organised and easily understandable format, aiding in communication and decision-making.
Structured Reporting
Unstructured data is a broader term that encompasses any data lacking a predefined structure. Unstructured data may include both qualitative and quantitative information.
Unstructured
Qualitative
VS
Qualitative data refers to non-numeric information and can be unstructured, structured, or semi-structured.The difference between qualitative and unstructured data is the focus is of the information rather than its organization.
Unstructured data analysis equips professionals to adapt to diverse data formats, preparing them for the dynamic nature of data in the modern world.
Adapting to Diverse Data Formats
Analysts develop interdisciplinary skills, combining knowledge from fields like linguistics, computer vision, and audio processing to tackle the challenges of unstructured data.
Interdisciplinary Skills Development
Communicating findings from unstructured data analysis requires effective communication skills, enabling analysts to convey insights to diverse audiences.
Enhancing Communication Skills
Structured data can be processed efficiently using various data processing and analysis tools.This quick and effective data processing support tasks such as sorting, filtering and data aggregation.
Efficient Processing
Unstructured data often exists in large volumes and can be generated rapidly.Recognizing the high volume and velocity informs decisions about storage, processing capabilities, and the scalability of systems handling unstructured data.
Volume and Velocity
High-quality structured data is essential for compliance with regulatory requirements and governance standards. It ensures that organizations can trust their data for audit and reporting purposes.
Compliance and Governance
Structured data often involves relationships between different entities. Knowing these relationships is important for maintaining data integrity and designing effective database structures.
Data Relationships
Analysing unstructured data requires specialised techniques, such as text mining, image recognition, and audio processing.Knowing which techniques are appropriate for different types of unstructured data is essential for conducting meaningful analyses and extracting valuable insights.
Analysis Tecniques
Unstructured data quality ensures that data is interpreted in the correct context, allowing for a more nuanced understanding of information and avoiding misinterpretations.
Contextual Understanding
Structured data allows for the aggregation of information through functions like sum, average, and count, providing summarized insights into various aspects of the dataset.
Aggregated Insights
Quantitative are typically precise and accurate, allowing for rigorous analysis and numerical comparisons.
Quantitative
Qualitative data focuses on depth and context, providing rich insights, but may lack the precision associated with quantitative data.
Qualitative
VS
Unstructured data lack a clear organisational structure, often making it more challenging to manage and analyse.Understanding this complexity is crucial for implementing effective data preprocessing and cleaning techniques before analysis.
Complexity and Lack of Organisation
Quantitative analysis techniques include machine learning algorithms and mathematical models to identify patterns, trends, and relationships within structured data.
Quantitative
Qualitative analysis techniques include text mining, sentiment analysis, or natural language processing. Techniques involve identifying patterns, themes, and sentiments in unstructured data.
Qualitative
VS
Consistent and reliable structured data fosters trust among stakeholders, customers, and partners. It enhances the credibility of the information presented by the organisation.
Customer Trust
Using structured data allows for efficient querying, enabling quick and precise retrieval of information based on specific criteria.
Efficient Querying
Users can perform ad hoc queries on structured data, allowing for on-the-fly analysis and exploration of specific aspects without the need for predefined reports.
Ad hoc Queries
Understanding ethical considerations in unstructured data analysis is essential, promoting responsible use of data and addressing privacy and bias concerns.
Ethical Considerations
BI tools, designed for structured data, enable organizations to create meaningful visualizations, dashboards, and reports for data-driven decision-making.
Business Intelligence
What is Structured Data?
Extra Readings
What is Unstructured Data?
Structured vs. Unstructured Data: What’s the Difference?
What is Semi-structured data?
Non-mandatory
Ensuring the quality of unstructured data is essential for ethical considerations, particularly when dealing with sensitive information, privacy concerns, and potential biases in algorithms.
Ethical Considerations
Quality unstructured data facilitates effective exploration and understanding of diverse information sources, promoting innovation, creativity, and the discovery of new insights.
Comprehensive Data Exploration
Unstructured data sources, such as social media or online content, are dynamic and continually evolving.Understanding the dynamic nature of unstructured data is essential for designing systems that can adapt to changes and emerging patterns over time.
Dynamic Nature
Reliable unstructured data quality is essential for image and video processing applications, including object recognition, computer vision, and facial recognition.
Enhanced Image and Video Processing
Reliable structured data is the foundation for data-driven innovation. It allows organisations to explore new technologies, implement advanced analytics, and stay competitive in a data-centric environment.
Data-driven Innovation
Quantitative visualisation is done through charts, graphs, histograms, and dashboards to represent numerical relationships and trends.
Quantitative
Quantitative visualisation is done using techniques such as topic modeling or sentiment heatmaps to represent patterns and trends in textual or non-numeric data.
Qualitative
VS
Structured data analysis is fundamental for developing and applying predictive models, supporting forecasting and decision-making based on historical patterns.
Predictive Modeling
Unstructured data may contain ambiguous or context-dependent meanings.Recognizing semantic ambiguity is essential for deploying advanced natural language processing and machine learning techniques to infer meaning from unstructured textual data.
Semantic Ambiguity
Structured data is often queried using structured query languages (SQL) or similar tools, allowing for precise retrieval of information. This facilitates analytical processes, making it easier to extract insights, generate reports, and perform complex queries.
Querying and Analysis
Unstructured data can come from a wide range of sources, including social media, documents, images, and sensor data.Recognising the varied sources helps in understanding the context in which the data is generated and making informed decisions about its usability and reliability.
Varied Sources
Addressing data quality issues proactively reduces the costs associated with data errors, corrections, and potential business disruptions caused by inaccurate information.
Reduced Costs
The date represents the date of the sales transaction. The products describes the product being sold (categorical data). The units sold quantifies the number of units sold for each product on a specific date (quantitative data). The revenue quantifies the revenue generated from the sales for each product on a specific date (quantitative data).This dataset is structured because it is organized into rows and columns with clear headers, making it easy to understand and analyze. The quantitative information (Units Sold and Revenue) is numerical, allowing for mathematical calculations and statistical analysis..
Quality unstructured data enables organisations to extract meaningful insights, sentiments, and patterns from textual, visual, or auditory information, contributing to more informed decision-making.
Insightful Analysis
High-quality unstructured data supports accurate speech recognition systems, enhancing the performance of voice-activated technologies and virtual assistants.
Improved Speech Recognition
Unstructured data does not adhere to a predefined schema, making it challenging to interpret systematically. Recognizing the absence of a fixed structure is vital for devising flexible analysis approaches that can adapt to the ever-changing nature of unstructured data.
No Fixed Schema
Unstructured data quality ensures that content discovery and search mechanisms yield relevant and contextually accurate results, improving the user experience in content-rich environments.
Meaningful Content Discovery
Quality unstructured data supports decision-makers by providing a more comprehensive and nuanced view of complex situations, enabling them to make better-informed choices.
Informed Decision Making
High-quality structured data ensures that decisions based on the data are accurate and reliable, contributing to better business outcomes and strategies.
Accurate Decision Making
Quantitative data can be processed efficiently using automated tools and algorithms, enabling quick analysis of large datasets.
Quantitative
Qualitative data analysis may be time-consuming, especially with large volumes of unstructured data, and often requires qualitative expertise.
Qualitative
VS
Structured data is organised, typically residing in databases with predefined schemas. It follows a clear structure, making it suitable for efficient storage and retrieval.
Structured Data:
Unstructured Data:
Unstructured data lacks a predefined data model and is often found in diverse formats. It requires more advanced techniques for analysis, such as natural language processing.
Semi-structured Data:
Some data does not neatly fit into the structured or structured categories. These data are typically converted into structure data for easier analysis.
Structured Data
Sam accessed the company's financial records, customer transactions, and inventory lists were neatly organized in databases and spreadsheets. Sam could easily extract insights, generate reports, and guide the CEO in making data-driven decisions. The structured data allowed Sam to navigate through the numbers with precision and accuracy.
Structured data analysis aids in maintaining regulatory compliance by providing a structured and auditable trail of data interactions, which is essential for governance and reporting..
Regulatory Compliance
Quality structured data contributes to a better understanding of customer preferences and behaviour, leading to improved products, services, and overall customer satisfaction.
Improved Customer Experience
Insights derived from unstructured data contribute to informed decision-making, especially in fields like marketing, where understanding customer sentiments is crucial.
Enhancing Decision Making
Mastery of advanced NLP techniques empowers analysts to comprehend and interpret textual data, providing a deeper understanding of language nuances.
Advanced NLP and Language Processing
Structured data analysis heavily relies on SQL, providing a standardised language for interacting with relational databases, executing queries and aggregating information.
Structured Querying Languages
The data is unstructured because each review is presented in a free-text format without a predefined structure. The information is qualitative, as it represents opinions, sentiments, and detailed feedback from customers. Unstructured data like this can be challenging to analyze using traditional structured data analysis methods but can be valuable for gaining deeper insights into customer experiences and preferences. Natural Language Processing (NLP) techniques are often used to extract meaningful information from unstructured textual data like customer reviews.
Structured data supports statistical analysis methods, facilitating the exploration of relationships, trends, and patterns within the dataset.
Statistical Analysis